Overcoming Challenges in ANOVA Testing Assignments: A Comprehensive Guide for Students
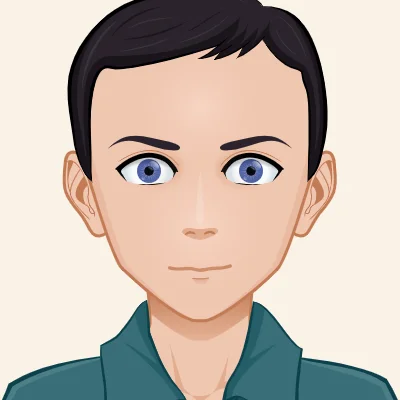
Welcome to our comprehensive guide on complete in ANOVA testing assignments. ANOVA, or Analysis of Variance, is a statistical technique used to analyze differences between multiple groups. While ANOVA is a powerful tool, students often face various difficulties when tackling ANOVA testing assignments. In this guide, we will provide you with practical strategies, tips, and expert advice to help you with ANOVA testing and excel in your assignments. From understanding the core concept of ANOVA to interpreting complex statistical output, we'll cover it all.
We understand that real your ANOVA assignment can be intimidating, especially for those new to statistics. That's why we have compiled this comprehensive guide to address the most common challenges students face. You'll gain a deeper understanding of ANOVA, learn how to choose the appropriate ANOVA test and formulate clear research questions and hypotheses. We'll delve
Interpreting statistical output and understanding concepts like effect size and post hoc tests are often stumbling blocks for students. Rest assured, we'll guide you through these topics, providing detailed explanations and examples. Time management, seeking help and resources, and overcoming fear and anxiety are crucial aspects of completing ANOVA assignments. We'll provide tips on effective time management, utilizing available resources, and adopting a positive mindset to tackle challenges head-on.
Throughout this guide, we'll emphasize the importance of practice and continuous learning. Engaging with real-world examples and staying updated with the latest research in statistical analysis will enhance your ANOVA skills. Join us on this journey to overcome challenges in ANOVA testing assignments and elevate your statistical prowess.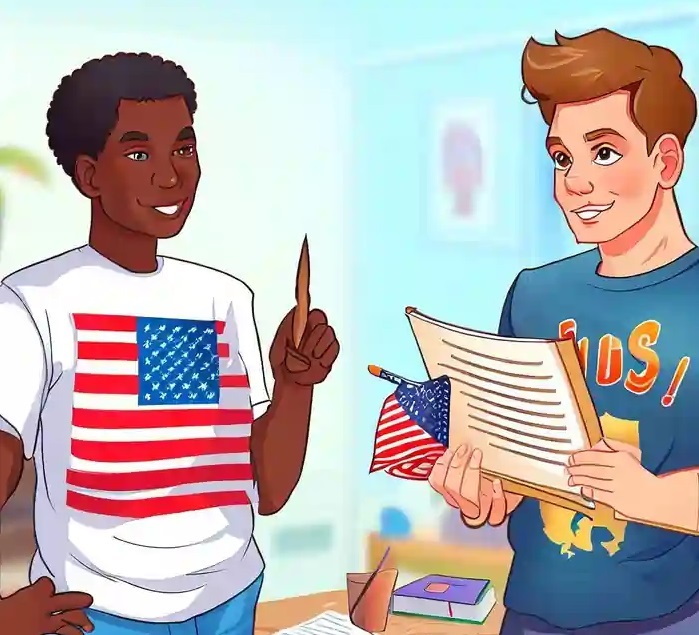
Understanding the ANOVA Concept
ANOVA is a statistical method for examining how the means of two or more groups differ from one another. Students must have a solid understanding of the fundamental idea behind ANOVA assignments to complete them. ANOVA compares between-group variability to within-group variability, which measures variation within and between groups. We can use ANOVA to analyze these differences and determine whether the observed variation is the result of meaningful group differences or random chance.
Students should become familiar with the fundamentals of statistical inference, such as hypothesis testing, p-values, and confidence intervals, to understand the concept of ANOVA. They should be aware of the basic tenets of ANOVA, including the independence of observations, homogeneity of variances, and normality of data.
Choosing the Correct ANOVA Test
ANOVA tests come in a variety of forms, each of which is appropriate for various research questions and experimental setups. One-way ANOVA, two-way ANOVA, and repeated measures ANOVA are the most popular varieties. While; two-way ANOVA is appropriate for examining the effects of two independent variables on a dependent variable, one-way ANOVA is used when comparing means across multiple independent groups. On the other hand, repeated measures ANOVA is utilized when the same variable is measured numerous times under various circumstances. A thorough analysis of the research question and experimental design is necessary to select the appropriate ANOVA test. The number of independent variables, the degree of the dependent variable's measurement, and the nature of the experimental conditions should all be taken into account by the students.
The creation of the research question
For ANOVA assignments, developing a precise and narrowly focused research question is crucial. The analysis should be guided by the research question, which will also help choose the right ANOVA test. Students frequently struggle to formulate a research question that fits the assignment's goals and is suitable for ANOVA analysis. Students should take into account the population being studied, the relevant variables, and the anticipated results when formulating the research question. The research question should be precise, quantifiable, and consistent with the study's main goals.
Gathering and Preparing Data
For an ANOVA analysis to be useful, the data must be of high quality and be relevant. It may be difficult for students to gather information from reliable sources and guarantee its integrity. Additionally, time-consuming and error-prone tasks like data cleaning, coding, and organization can be involved in data preparation. Students should carefully plan their data collection process to meet these challenges. They must choose trustworthy sources, take sample size requirements into account, and use the right data collection techniques. It's crucial to validate the data for accuracy, look for missing values, and, if necessary, transform variables. To ensure accurate and trustworthy ANOVA results, careful data preparation is required.
Conditions for ANOVA
For accurate and reliable results, the ANOVA relies on several presumptions. These presumptions cover the independence of observations, homogeneity of variances, and normality of data. According to the normality assumption, each group's data should have a normal distribution. The reliability of the p-values and confidence intervals may be impacted by a violation of this premise. Students should use graphical tools like histograms or statistical tests like the Shapiro-Wilk test to evaluate the normality of their data.
Homogeneity of variances assumes that the variances between groups ought to be roughly equal. This assumption's violation might affect how accurate the F-statistic and subsequent hypothesis tests are. Students can evaluate the homogeneity of variances using statistical tests like Levine's test or visual methods like boxplots. The premise behind independent observations is that the data points are unaffected by one another. Techniques for experimental design or random sampling are frequently used to accomplish this. Potential dependencies in the students' data should be taken into account and accounted for in the analysis. For an accurate ANOVA analysis, it is essential to comprehend and validate these assumptions. Alternative techniques, such as non-parametric tests or the transformation of variables, may be needed if the assumptions are broken.
Understanding Statistical Software Results
The majority of ANOVA analyses are carried out with statistical programs like SPSS or R. Although these tools offer quick and accurate calculations, students might have trouble comprehending and interpreting the results these programs produce. Various statistical measures and test results are frequently included in the output of statistical software. It might display the F-statistic, degrees of freedom, p-values, effect sizes (like partial eta-squared), and the outcomes of post hoc tests, for instance. To get useful information from the output of the software, students should become familiar with the interpretation of these measures.; For instance, the F-statistic calculates the proportion of variance within and between groups. A high F-value denotes the presence of significant group differences. The degrees of freedom plays a crucial role in establishing the F-distribution's critical values. P-values indicate the likelihood that results could have been observed solely by chance, with lower p-values indicating more compelling evidence against the null hypothesis. The practical significance of the observed differences is revealed by effect sizes. When the overall ANOVA test is significant, post hoc test results can be used to pinpoint specific group differences.
Post-hoc analyses and numerous comparisons
Post hoc tests are used to pinpoint specific group differences when an ANOVA shows a significant difference between the groups. Multiple pairwise comparisons between groups are possible using post hoc tests, which aid in identifying which particular groups are significantly different from one another. There are several post hoc tests available, including Schefft's test, Bonferroni, and Tukey's Honestly Significant Difference (HSD). When dealing with multiple comparisons, students may find it difficult to decide which post hoc test to use and how to interpret the results correctly. Students should think about things like the desired control of Type I error rate, the test's underlying assumptions, and the type of data when selecting an appropriate post hoc test. They should carefully analyze the data, taking into account the effect sizes, adjusted p-values, and confidence intervals.
Calculating Effect Size
The ANOVA test's effect size quantifies the size of the differences that were found. It offers important details about the findings' relevance to daily life. ANOVA frequently employs Cohen's d, Eta-squared, or Omega-squared as measures of effect size. When comparing two groups, Cohen's d, which measures the standardized mean difference between groups, is especially helpful. Omega-squared offers a more precise estimate of effect size in situations with unequal group sizes, whereas Eta-squared measures the percentage of variance explained by the independent variable(s). Accurately calculating and interpreting effect sizes may be difficult for students. It is crucial to take into account the study's setting, the scope of the measurements, and the particular effect size measure being employed. Researchers can assess the practical significance of their findings beyond statistical significance by interpreting effect sizes.
Developing the Hypotheses
For ANOVA assignments, it's essential to develop precise, testable hypotheses. The research question should be in line with the hypotheses, which should also direct the analysis. Developing hypotheses that accurately reflect the research question and can be examined using ANOVA techniques is a challenge for many students The alternative hypothesis (Ha) in an ANOVA typically contends that at least one group differs from the others while the null hypothesis (H0) typically asserts that there are no differences between the groups. The way that students formulate their hypotheses should enable them to conduct hypothesis tests and come to conclusions after the analysis.
The following are some possible hypotheses for a one-way ANOVA comparing the means of three groups
H0: (There is no difference in the means of the three groups) 1 = 2 = 3
Ha! One man stands out from the others at least.
Students can stay focused by writing concise, clear hypotheses, which also makes it easier to interpret the findings.
Carrying Out a Power Analysis
Power analysis is essential for figuring out the sample size needed for ANOVA testing to find a significant effect. The term "power" describes a statistical test's capacity to identify an effect, if one exists. Conducting power analyses can be difficult for students because it requires intricate calculations and an understanding of statistical power concepts.
Students should think about the desired power level (typically 80% or 90%), the effect size they want to detect, the significance level (alpha), and the anticipated sample sizes when performing a power analysis. Students can use power analysis to decide whether their sample size is sufficient to find meaningful effects and to help them make decisions about the viability of their study.
Power analysis can be aided by several software programs and online calculators, which makes the process more approachable for students.
Results Represented Graphically
Understanding and readability can be improved by using the right graphs and visualizations to present the results of the ANOVA test. Selecting and making effective graphs to represent the findings may prove challenging for students. The type of graphic representation depends on the research question and the type of data. The means and variations of a group are frequently shown using bar charts or boxplots. To see how two independent variables affect the dependent variable, use an interaction plot. To effectively communicate the key findings of the ANOVA analysis, students should make sure that the chosen graph accurately depicts the data. To facilitate interpretation, there should be clear labeling, suitable scales, and instructive captions. The patterns, trends, and differences in the data can be easier for readers to understand when they are represented graphically. Main Effects and Interactions Interpretation
Main effects (differences between groups) and interactions (how the effects of one factor depend on the levels of another factor) are both investigated by ANOVA analysis. Since this requires a thorough understanding of both the research question and the data, students may find it challenging to interpret and explain the main effects and interactions accurately. The overall variations between groups or levels of an independent variable are represented by main effects. They represent each factor's effect without regard to other factors. Conversely, interactions show that the impact of one independent variable is influenced by the values of another independent variable. When interpreting interactions, it is important to take into account the study's context as well as the direction and size of the effects. The distribution of means and interactions should be carefully examined by students in the ANOVA output. They should think about how the main effects and interactions relate to the research question and whether they are statistically significant. The main effects and interactions are explained in detail to give the ANOVA analysis more depth and clarity.
Managing Missing Data
During an ANOVA analysis, missing data can present problems. The absence of observations for particular variables or cases is referred to as missing data. To ensure the integrity and validity of their findings, students must learn methods for handling missing data, such as imputation or conducting sensitivity analyses. Various factors, such as non-response, data entry mistakes, or dropout rates in longitudinal studies, can result in missing data. Results may be skewed or ineffective if missing data is ignored or cases with missing values are excluded. To properly handle missing data, students should investigate techniques like mean imputation, multiple imputations, or maximum likelihood estimation. Imputation methods substitute missing values for estimated values based on the information at hand. By examining various hypotheses and scenarios, sensitivity analyses can assist in determining the effect of missing data on the outcomes. Missing data must be dealt with carefully, and the solution must be disclosed transparently.
Management of Time
Assignments involving ANOVA can take a lot of time, especially when dealing with complicated datasets or numerous factors. Because they frequently have trouble managing their time, students may find it challenging to finish the assignment within the allotted time. Students should make a thorough plan and schedule for their ANOVA assignment to efficiently manage their time. The tasks can be divided into smaller, more manageable chunks, which can aid in properly allocating time. Setting realistic deadlines is crucial, allowing enough time for the gathering, analysis, interpretation, and writing of the data. Students can stay on track by setting priorities and asking for help when they need it. To complete assignments on time while maintaining the accuracy and quality of the ANOVA analysis, time management skills are essential.
Theory and Application in Balance
Assignments for ANOVA must strike a balance between theoretical ideas and real-world applications. Integrating statistical theory with the actual application of ANOVA analysis may be challenging for students. Students should be able to apply the fundamental statistical concepts to actual data and research questions in addition to having a solid understanding of the underlying statistical principles. Making connections between the theoretical ANOVA concepts and the specific context of the assignment is crucial, as is using the analysis to derive meaningful conclusions. Students should actively interact with the data, look for examples or case studies pertinent to their research question, and critically consider the practical implications of their findings to strike a balance between theory and application. This strategy improves the assignment's quality and the general understanding of ANOVA.
Using Resources and Assistance
When given difficult ANOVA assignments, students should ask their professors, peers, or online resources for help. Finding trustworthy sources of assistance and utilizing them wisely can be difficult enough Students should ask their teachers or teaching assistants for advice on their initiative. They can make appointments to discuss analysis techniques, clear up any questions, or get feedback on their progress. Students can be a great resource for group projects and in-depth discussions of difficult ANOVA concepts. Online sources like textbooks, scholarly journals, or statistical discussion boards can offer additional assistance. Students should assess the authority and applicability of the sources they use critically
The difficulty of ANOVA assignments can make students anxious and afraid of failing. Creating strategies to control these feelings and keep a positive outlook throughout the assignment is essential. The first step is to identify and acknowledge these emotions. Students should be aware that feeling anxious when tackling difficult tasks is common. They can use techniques like segmenting the assignment into smaller tasks, taking care of themselves, asking their peers for support, and acknowledging small victories along the way. Students can also get over their fears and anxieties by adopting a growth mindset, which emphasizes constant learning and improvement rather than fixed abilities. It is possible to develop a positive attitude toward the assignment by accepting the difficulties of ANOVA assignments as opportunities for development and learning.
Practice and Ongoing Education
In the end, practice and ongoing education are the keys to mastering ANOVA testing. Students should perform practical data analysis, look for opportunities to use ANOVA techniques in practical settings and keep up with the most recent developments in statistical analysis. Students' understanding of the concepts and their capacity to correctly interpret the results can be improved by having them practice ANOVA analysis with a variety of datasets and research questions. To gain experience, students can explore datasets that are readily available online or create their data. Additionally, reading pertinent literature, taking part in workshops or webinars, and participating in statistical forums can expose students to various viewpoints and broaden their knowledge. Continuous learning entails remaining inquisitive, raising queries, and looking for chances to broaden one's statistical knowledge beyond that of ANOVA. This method helps students develop a thorough understanding of statistics and improves their capacity to complete difficult ANOVA assignments.
Conclusion
In conclusion, students may find it difficult to complete ANOVA testing assignments, but with the right strategies and direction, you can overcome the typical difficulties and achieve success in your statistical analysis. You have received useful advice, professional counsel, and a road map to help you navigate the complexities of ANOVA in this thorough guide.
In this guide, we have looked at the 18 typical issues that students run into when completing a challenging ANOVA testing assignment. We have covered each topic in great detail, from comprehending the concept of ANOVA to interpreting statistical output, handling missing data, and time management.