Exploring the 8 Most Important Kinds of SPSS Assignments Used in Teaching at Universities
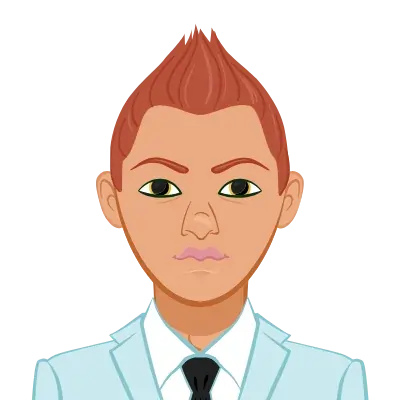
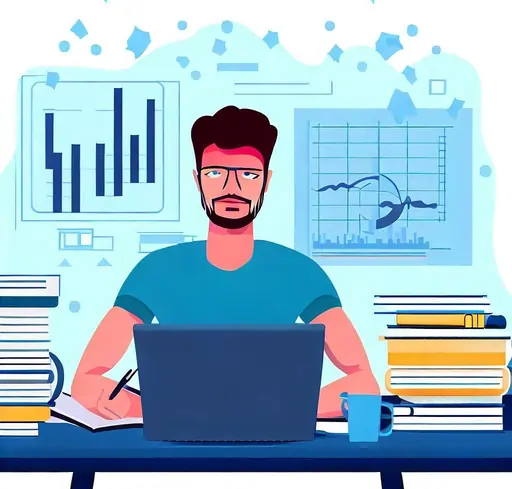
- Statistically Descriptive:
- Statistical Inference:
- Relationship Analysis:
- Analysis of Regression:
- Component Analysis:
- Crowd Analysis:
- Nonparametric Evaluations:
- Survival Evaluation:
The main goal of descriptive statistics assignments in SPSS is to summarize and analyze data in order to better understand its fundamental properties. Calculating metrics like mean, median, mode, range, and standard deviation is a common task for these assignments. Students can learn about the central tendency and variability of their data by computing these statistical measures.
Descriptive statistics assignments in SPSS also introduce students to graphical representations in addition to numerical summaries. In order to understand data distributions and proportions visually, they learn how to make and interpret histograms, bar charts, and pie charts. These representations aid in finding trends, outliers, and patterns in the data.
The basis for more sophisticated statistical analyses is a descriptive statistics assignment. By becoming adept at these methods, students develop the knowledge and abilities required to accurately describe and present their data in a clear and concise manner, laying the foundation for further investigation.
Assignments involving inferential statistics in SPSS are designed to make inferences about a population from a sample. These tasks involve performing analysis of variance (ANOVA), estimating confidence intervals, and testing hypotheses.
Students create alternative and null hypotheses and use statistical tests to assess the evidence in support of each one. Hypothesis testing is a fundamental concept in inferential statistics. Students can analyze their data using a variety of tests offered by SPSS, including t-tests and chi-square tests, to decide whether to accept or reject their hypotheses.
Another crucial component of inferential statistics is estimating confidence intervals. Students gain knowledge of how to compute intervals that offer a variety of believable values for population parameters. These intervals offer important clues about the accuracy and dependability of sample estimates.
Assignments involving analysis of variance (ANOVA) teach students how to compare means across various groups. To ascertain whether there are statistically significant differences between group means, they are taught how to conduct ANOVA tests. Students can investigate how categorical variables affect continuous outcomes in this way.
Students gain critical thinking abilities and the capacity to draw reliable conclusions from sparse data by working on inferential statistics assignments, which enhances their comprehension of the entire scientific research process.
In SPSS assignments involving correlation analysis, relationships between two or more variables are looked at. Students gain knowledge of how to compute and interpret correlation coefficients, such as Pearson's correlation coefficient.
The strength and direction of the linear relationship between two variables are expressed in terms of correlation coefficients. They fall between -1 and +1, with -1 denoting a perfect negative correlation, +1 denoting a perfect positive correlation, and 0 denoting no correlation. Students gain understanding of how variables are connected and whether they move in unison or opposition through these assignments.
In assignments involving correlation analysis, interpreting the significance of correlation coefficients is essential. In order to determine whether the relationships seen in their data are likely to occur in the general population, students learn to determine whether the observed correlations are statistically significant.
Students have the chance to investigate the limitations of correlation as a statistical measure through correlation analysis assignments. They discover the significance of taking into account additional elements, such as causality, confounding variables, and outliers, in order to prevent erroneous or misleading interpretations.
The ability of students to recognize and quantify relationships between variables is improved by correlation analysis assignments in SPSS, which enables them to make defensible decisions and predictions based on data patterns.
Students can investigate the relationships between dependent and independent variables through regression analysis assignments in SPSS. Regression model fitting, residual analysis, and coefficient interpretation are all part of these assignments.
Regression models are a tool that students learn to use to calculate the effect of one or more independent variables on a dependent variable. Regression techniques are covered, including multiple, logistic, and linear regression, each of which is appropriate for a particular research scenario.
Regression analysis assignments often involve the analysis of residuals. The discrepancies between the values that were observed and those that the regression model predicted are represented by residuals. Regression assumptions, such as the normality of the residuals, are evaluated by students in order to determine the validity of the model.
A crucial skill gained from these assignments is the ability to interpret regression coefficients. Based on the coefficients, students learn to identify the direction and size of the relationships between variables. To understand regression analysis better, they also investigate ideas like statistical significance, confidence intervals, and multicollinearity.
Students develop crucial analytical skills that enable them to recognize the variables influencing a specific outcome and make predictions based on their findings by working on regression analysis assignments in SPSS. These abilities are transferable to a variety of industries, such as the social sciences, business, and healthcare.
In SPSS, factor analysis assignments look for underlying factors that contribute to the patterns of observed variables. Students gain knowledge of factor rotations, factor extraction, and factor interpretation.
When working with complex data sets containing lots of variables, factor analysis is especially helpful. By locating latent variables that account for the relationships between observed variables, it aids in reducing the dimensionality of the data. Students improve their understanding of the underlying constructs or dimensions represented in their data by categorizing related variables into factors.
Finding the variables that are most strongly associated with each factor is necessary for factor extraction. Students study various extraction techniques, such as principal component analysis (PCA) and principal axis factoring (PAF), and they investigate the standards for deciding how many factors to keep.
Factor rotations are designed to make the factor structure easier to understand. The various rotation techniques, such as varimax and obliging rotation, are covered in class, and students gain an understanding of how rotations alter the distribution of factor loadings.
In factor analysis assignments, interpreting factor loadings is a crucial step. The strength and direction of the relationships between the variables and the factors are indicated by the loadings. Students learn which variables contribute most to each factor and how they are related to the underlying construct by examining loadings.
Students can find hidden patterns, simplify complex data, and comprehend the underlying structures of their data sets by using factor analysis assignments in SPSS. These abilities are useful in industries like psychology, market research, and survey analysis where latent variable identification and data reduction are crucial.
In SPSS assignments involving cluster analysis, similar cases or objects are grouped according to their attributes. In order to find natural groupings within data, students learn how to use clustering techniques like k-means clustering or hierarchical clustering.
With the help of the unsupervised learning method of clustering, students can examine the patterns and resemblances in their data without the need for predefined groups. Students can recognize homogeneous subgroups that have similar traits by allocating cases to clusters.
By minimizing the sum of squares within the cluster, cases are grouped using the popular K-means clustering technique. In order to gain insights into data patterns, students learn to choose the ideal number of clusters and interpret the outcomes.
Creating a hierarchy of clusters using linkage techniques like Ward's method or complete linkage is known as hierarchical clustering. Dendrogram interpretation and determining the ideal number of clusters based on visual inspection or statistical criteria are skills that students acquire.
Students learn how to recognize significant subgroups within their data sets by working on cluster analysis assignments. They discover how to investigate the traits that characterize each cluster and comprehend the usefulness of such classifications. Numerous fields, including market segmentation, customer profiling, and social sciences, use cluster analysis.
In SPSS, nonparametric test assignments are intended for circumstances in which the data do not adhere to the assumptions of parametric tests. Tests like the Mann-Whitney U test, Kruskal-Wallis test, and Wilcoxon signed-rank test are taught to students.
When analyzing ordinal or non-normally distributed data or when parametric test premises like independence or equal variances are broken, nonparametric tests are useful. These tests offer substitute statistical techniques that do not rely on particular distributional hypotheses.
In order to determine whether there is a significant difference between two related variables, the Wilcoxon signed-rank test compares paired observations. This analysis can be extended to compare three or more independent groups using the Kruskal-Wallis test. Two independent groups are compared using the Mann-Whitney U test.
Students gain knowledge of how to choose the best test based on the characteristics of their data and research questions through nonparametric test assignments. They gain knowledge about how to carry out and analyze these tests, including knowledge of effect sizes and results reporting.
Nonparametric tests give students flexibility and robustness in data analysis, ensuring that statistical conclusions are accurate even in difficult situations. Students can approach a wider range of research questions by mastering nonparametric tests in SPSS and expanding their analytical toolbox.
Analyzing time-to-event data, such as the time to failure or the occurrence of an event, is a requirement for survival analysis assignments in SPSS. Students gain knowledge of how to calculate log-rank tests, estimate survival curves, and read hazard ratios.
In the social and medical sciences, survival analysis is frequently employed to study phenomena like disease progression, mortality rates, and customer churn. Students can investigate how outcomes are time-dependent and look at what factors affect survival or the occurrence of events.
Kaplan-Meier curves, which are frequently used to represent survival curves, show how likely an event is to occur over time. In addition to determining median survival times and contrasting survival distributions between groups, students learn how to estimate and interpret these curves.
Students can assess whether significant differences in survival curves between groups exist using log-rank tests. These tests enable comparisons between categorical variables and shed light on how various variables affect survival outcomes.
The relative risk of experiencing an event between two groups is measured by hazard ratios. The interpretation of hazard ratios and their implications for event occurrence or survival are two skills that students acquire.
Students gain experience handling time-to-event data and a deeper comprehension of the variables that affect outcomes over time by working on survival analysis assignments. Clinical trials, epidemiology, and the social sciences are just a few of the fields where these analytical methods are put to use in real-world situations.
Conclusion:
In conclusion, incorporating SPSS assignments into university instruction provides students interested in a career in data analysis and research with an essential stepping stone. Universities make sure that students are ready to handle the demands of statistical analysis in the professional world by exposing them to a variety of SPSS assignment types.
The eight different SPSS assignment types covered in this blog post cover a wide range of statistical methods, such as regression analysis, hypothesis testing, and descriptive statistics. Each assignment forces students to put their understanding of SPSS and statistical ideas to use in practical situations, fostering a deeper comprehension of statistical analysis techniques.
Students gain technical proficiency in using SPSS as well as the critical thinking abilities necessary to make wise decisions based on data by completing these assignments. They gain the ability to navigate through large, complex data sets, spot relationships and patterns, and reach meaningful conclusions. Additionally, the practical knowledge acquired through these assignments boosts students' self-assurance in using SPSS as a potent tool for statistical analysis.
Knowledge of SPSS and statistical analysis is highly valued in the cutthroat job market. Students who perform well on these SPSS assignments have a distinct advantage because they can show that they are capable of handling difficult data challenges in the real world. SPSS assignments continue to be a key component in fostering the next generation of knowledgeable statisticians and data analysts as universities continue to emphasize the value of practical application.
Therefore, the variety of SPSS assignments covered in this blog will give you useful insights and prepare you for the exciting world of data analysis, whether you are a student starting out on a statistical analysis journey or an academic looking to expand your knowledge.